Predictive analytics and its relationship with big data
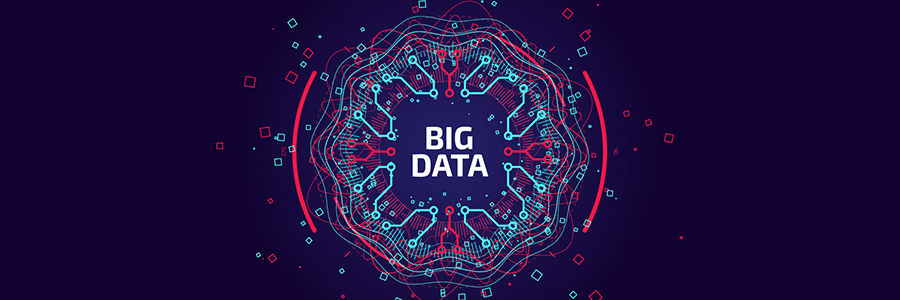
The practical result of BI (Business Intelligence) and Big Data is Practical Analytics. What will you do when the date of your business starts to stagger? The business applications in today’s era are scraping in mountains of a new market, customers, real-time app, social listening, and performance date of the product. All of the information can be leveraged with the help of predictive analytics. Also, you can gain access to new tangible insights, and stay way ahead of the competition in your industrial niche.
Predictive analytics is made use of by companies and various organizations in many different techniques. They use in for data mining, predictive marketing, artificial intelligence, and machine learning as well. This is mainly done to unveil the new patterns of statistics and make use of certain algorithms in optimizing the business processes.
The method is particularly based on one agenda in which the computers make use of the data that were determined from past behavior and how can the businesses make use of this information to promote better functioning. Also, this is used to determine the way businesses work. However, before we indulge in the topic where predictive analytics is used by companies to save money, time, and get an edge over the market; it’s important that we understood what predictive analytics is and how does it function?
What do you understand by predictive analytics?
Predictive analytics cannot be regarded as the concept which is completely black-and-white. Also, it cannot be regarded as a discrete feature which can only be decoded by modern-day database managers. A bunch of statistical techniques and data analysis techniques is rolled up under the same banner. The core technique of predictive analytics is regression analysis. It is used to determine values that are of multiple variables that are correlated on the basis of disproving or approving a certain assumption.
The ones possessing expertise in the field of predictive analytics say that it is all about identifying the patterns of the data deduced for the determination of project probability. Also, you need to know that predictive analytics is solely based on probabilities and not on absolutes. It doesn’t work in the same way as traditional analytics, where the analyst is already aware of the type of data that needs to be used for retrieving the desired output.
Along with the usage of regressive analysis, predictive analytics makes use of ML and data mining progressively. Data mining’s definition matches up to the word. In this, a huge amount of data is taken into consideration to examine their patterns and discover new pieces of information. ML techniques are making use of sifting pickaxes and pans to settle on data nuggets made up of gold.
Through the innovations used up in Machine Learning in this era, deep learning algorithms and neural networks can process the set of unstructured data way faster than the conventional researcher or data scientist. And, the result obtained shall have superior accuracy as compared to the manual work done. The big change that is advancing towards predictive analytics is not just the initiation of Artificial Intelligence and Machine Learning, but the techniques are not just being used by the data scientists alone.
With the help of data visualization and BI tools, organizations are making the Big Data tools more efficient, easier to use, and more accessible than ever before. Everyday business users are making use of ML data analysis tools for the purpose of self-services. The salesperson of the business is making use of such tools to analyze lead data, while the executive is trying to decipher the trends that are ongoing in the market currently, the marketing manager of social media is gauging on the demographics of the social trends and the followers as well to make sure that the campaign reaches up to the targeted audience, and the representative of customer services tries to find out about the pain points experienced by the customer.
These cases that are mentioned are just the iceberg’s tip, in which predictive analytics can be efficiently used. Predictive analytics is changing the way the world used to work and engaging new techniques for the betterment of different industrial niches. However, you shouldn’t live with the idea that predictive analytics will be able to depict whether your to-be-launched product is going to return revenue of billions of dollars. Data even today remains the crucial part of an educated guess. But, the point is we are a lot more educated than we generally used to be.
Predictive Analysis can be used almost everywhere
As the landscape of Business Intelligence evolves, predictive analysis is having a good time in finding its way towards the roots of the used cases of businesses. There are various tools available in the market today that will help to make sense of the massive volumes of data imported by the businesses from several sources. Though the self-service tools that are available online aren’t equipped with the finest and most advanced form of predictive analytics tool yet, they make sure that the Big Data development services is converted into such an extent that their analysis becomes exceptionally easier.
The use of cases of predictive analytics in businesses can be classified under a broad spectrum. It is being used in the detection of POS (Point-Of-Sale) fraud, for automatically adjusting user context or digital content in order to drive conversions, and initiating customer services at a pro-active rate for risking the revenue services as well. In B2B marketing strategy, businesses make use of several tactics of predictive analytics to serve their customers with better services than the businesses that don’t make use of the same tool.
Here are three categories of B2B marketing strategy that lay the foundation for the implementation of more complex predictive analytics:
- Identification Models: In this case, the businesses that display a certain desired behavior, serve as the foundation of an identification model. The marketers can make use of this case to find the valuable prospects of sales in the earlier stages, prioritize the expansion of the existing accounts, unveil new marketing opportunities, and impart power to account-based marketing strategies by bringing the reasonably more receptive accounts to the surface for improving sales and spreading messages for marketing.
- Predictive Scoring: This is the most common way of B2B marketers into the world of predictive scoring as it helps to add mathematical and scientific dimensions to conventional prioritization, which relies completely upon experimentation, iteration, and speculation to drive weightings and criteria as well. This approach can be used effectively by marketers and sales to find about productive accounts with a faster approach and spend very less time on the accounts that are less likely to convert. This will basically be helpful in initiating targeted upsell or cross-sell campaigns.
- Automated Segmentation: Only the generic attributes of the B2B marketers have been segmented traditionally. And, it was done manually; therefore, the personalization that was applied was only to the campaigns that were of very high priority. However, the scenarios have changed now and the attributes can be used along with feed predictive algorithms, which can be effectively applied to the account records that support both augmented and intricate segmentation. Outbound communications with added relevant messages can be used by marketers to drive sales. It can also be used to initiate substantial conversation between the prospects and sales and deliver the content strategy more efficiently.